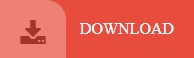
The resulting model is called "mixture distribution". Mixture designs are used to model the results of experiments where these relate to the optimization of formulations. The main difference comes from the model that is used. The analysis of a surface response design uses the same statistical and conceptual framework as linear regression. Remark: In contrast to this, screening designs aim to study the input factors, not the response value.įor example, suppose that an engineer wants to find the optimal levels of the pressure (x1) and the temperature (x2) of an industrial process to produce concrete, which should have a maximum hardness y. The family of surface response design is used for modeling and analysis of problems in which a response of interest is influenced by several variables and the objective is to optimize this response. In ANOVA, explanatory variables are often called factors. The main difference comes from the nature of the underlying model. Analysis of a screening designĪnalysis of a screening design uses the same conceptual framework as linear regression and variance (ANOVA). If the existing orthogonal designs in the knowledge base do not satisfy your needs, it is possible to search for d-optimal designs.
#ANOVA XLSTAT FULL#
Most common families like full factorial designs, Latin square and Placket and Burman designs are included. All existing orthogonal designs are available for up to 35 factors having each between 2 and 7 categories. Designs that are close to the design described by user input will be available for section without having to calculate for an optimal design. Orthogonal design tables are preferred, as the ANOVA analysis will be based on a balanced design. This tool integrates a large base of several hundred orthogonal design tables. There are other possible types of designs in order to take into account the limited number of experiments that can be carried out. But the number of necessary tests is often to large when using factorial designs. In general factorial designs are the most efficient for this type of study. The family of screening designs aims for the study of the effect of two or more factors. These analyses are included in the XLStat-Quality and XLStat-Premium packages.
#ANOVA XLSTAT TRIAL#
FeaturesĪ trial version of XLSTAT-Design of Experiments is included in the main XLSTAT download. XLSTAT-Design of Experiments contains all the classic experimental designs for screening factors such as factorial designs or Plackett-Burman designs as well as designs for optimization. XLSTAT can include in the model interactions and nested effects.XLSTAT-Design of Experiments is a complement to XLSTAT for those who want to design experiments in a structured way. We can drop this hypothesis when using the mixed model based approach. In repeated measures ANOVA we assume that the covariance matrix between the ys is spherical (for example, compound symmetry is a spherical shape). As measures are taken from the same subjects at different times, the repetitions are correlated. However, other hypotheses are necessary in the case of repeated measures ANOVA. The hypotheses used in ANOVA are identical to those used in linear regression: the errors εi follow the same normal distribution N(0,s) and are independent. Where y t i is the value observed for the dependent variable for observation i for measure t, k (i,j) is the index of the category of factor j for observation i, and e i is the error of the model. If p is the number of factors, the ANOVA model is written as follows: The exploratory variable is measured at different time or repetition. The main difference comes from the nature of the explanatory variables. Repeated measures Analysis of Variance (ANOVA) uses the same conceptual framework as classical ANOVA. Calculation in Repeated measures Analysis of Variance If the sphericity hypothesis is not rejected, between- and within-subject effects can be tested. For each measure, a classical ANOVA model is estimated, then the sphericity of the covariance matrix between measures is tested using Mauchly’s test, Greenhouse-Geisser epsilon or Huynt-Feldt epsilon. The principle of repeated measures ANOVA is simple. XLSTAT - Repeated Measures Analysis of Variance (ANOVA) Principles of Repeated measures Analysis of Variance
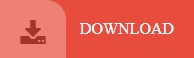